June 1, 2022 by Frederic Fol Leymarie
iSpot and AI: FASTCAT-Cloud
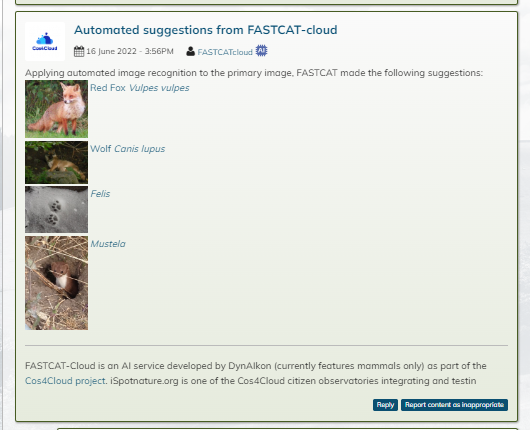
FASTCAT-Cloud is available under iSpot : it provides an initial analysis of your pictures or videos filtering relevant frames and recordings of wildlife activity to identify species names.
FASTCAT-Cloud is a website which facilitates uploading and analysing nature videos and pictures and provides information on relevant images and recordings of wildlife activity only.It also allows you to quickly identify species’ names with Artificial Intelligence (AI).
Read more at the iSpot Nature Forum